Shape-Based Coarse Graining
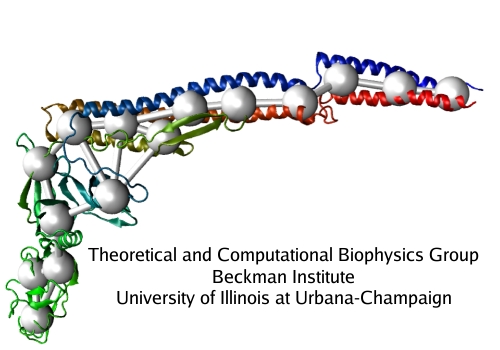
Shape-based coarse-graining is designed to model large-scale motions of macromolecular assemblies, representing proteins and other biomolecules with as few point-like particles as possible. Biomolecules, and proteins in particular, have a variety of shapes. Often, a single protein is comprised of both compact domains and disordered and elongated linkers or tails. The shape-based coarse-graining method enables one to model both compact domains and tails with equal accuracy, taking advantage of an efficient topology conserving algorithm originally developed for neural computations (Martinetz and Schulten, 1994). The placement of coarse-grain (CG) beads is performed using a self-organizing neural network, which adapts to the shape of the molecule to be represented. Implementation details can be found in Arkhipov, Freddolino, and Schulten, 2006.
Once CG beads are placed, they inherit the mass and charge of the groups of atoms they represent. Neighboring beads are connected by harmonic springs, while separate molecules interact through non-bonded forces (Lennard-Jones and Coulomb potentials). Interactions are parameterized on the basis of all-atom simulations and available experimental data. The solvent is modeled implicitly, using the Langevin equation to describe motions of beads, which allows one to introduce solvent viscosity. All-atom simulations or experimental data are used to parameterize the solvent model.
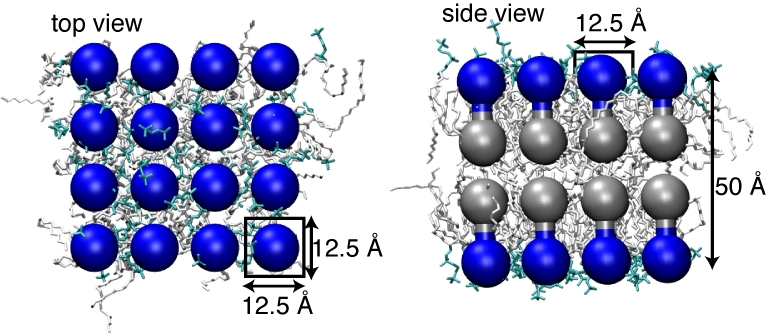
In addition to proteins, a shape-based CG model of lipids has been developed as well (Arkhipov, Yin, and Schulten, 2008). The lipid bilayer is modeled by two layers of CG beads for each leaflet: one for the lipid heads and one for the lipid tails, a head and a tail bead pair representing several lipid molecules together. The two beads within a head-tail pair are connected by a harmonic bond. This model has been successful in reproducing such properties of lipid bilayers as thickness, area per lipid, and bending rigidity. Also, disordered mixtures of shape-based CG "lipids" self-assembled into bilayer or other phases, with correct dependence on the concentration.
Simulations using the shape-based CG model demonstrate same performance scaling on parallel processors as that for an average all-atom simulation. With the dramatic reduction in the number of particles and with the simple solvent model used, the shape-based CG model achieves a 1,000-fold or more reduction in the number of particles to be simulated, and a 200-500-fold increase in the integration time step, allowing one to reach time scales of hundreds of microseconds and beyond.