Marcelo C. R. Melo
I am currently a postdoctoral researcher in the Machine Biology Group, at the University of Pennsylvania. My work focuses on exploring the Gut-Brain axis from a computational biology perspective, combining machine learning with systems- and structural-biology approaches.
Current Website: link here.
Email Address: melomcr at gmail dot com or melomcr at pennmedicine dot upenn dot edu
Education
- PhD in Biophysics (2019) - university of Illinois at Urbana-Champaign - USA
- Masters in Biophysics (2013) - Federal University of Rio de Janeiro - Brazil
- BS Biophysics (2011) - Federal University of Rio de Janeiro - Brazil
Advisor: Dr. Zaida Luthey-Schulten
Advisor: Dr. Pedro Geraldo Pascutti
Advisor: Dr. Pedro Geraldo Pascutti
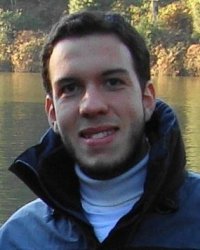