Carlos Cuellar Rodriguez
I am a PhD student at the University of Illinois at Urbana-Champaign and a graduate researcher at the Theoretical and Computational Biophysics Group (TCBG). My research work consists of developing and using deep learning approaches to study biophysical problems ranging from structural prediction, data analyses, and reaction coordinate prediction and selection. At the moment, I am focused on developing deep learning techniques for protein structure prediction capable of incorporating biophysical knowledge in their decision making. The overall driving aim of my research interests centers around elucidating the fundamental relationships between machine learning, statistical thermodynamics, and biophysics. The previously stated is viable given the nature and enormous size of data encountered in biophysics (from experiments to simulations, from proteomics to genomics). The field of biophysics is posed as prime ground to be enriched by deep learning techniques while serving as an avenue for development of new advancements in machine learning. Furthermore, I am also interested in machine learning aided coarse grained and molecular dynamics simulations of biophysical systems, using machine learning as a tool to explore and reevaluate fundamental statistical physics, and scientific leadership focused on the advancement of minorities in the field.
Home Department: Center for Biophysics and Quantitative Biology
Office Address: Beckman Institute, Room 3109, 405 N Matthews Ave, Urbana, IL 61801
Office Phone: +1 (217) 636-3315
Email Address: carlosc6@illinois.edu
Follow @CarlosRCuellarEducation
- Ph.D. in Biophysics and Quantitative Biology, The University of Illinois at Urbana-Champaign, Urbana, Illinois, 2025 (Expected)
- B.S. in Physics, The University of Texas at El Paso, El Paso, Texas, 2020
Advisor: Prof. Emad Tajkhorshid
Undergraduate Advisors: Dr. Jorge A Munoz, Dr. Lela Vukovic, and Dr. Jose Banuelos
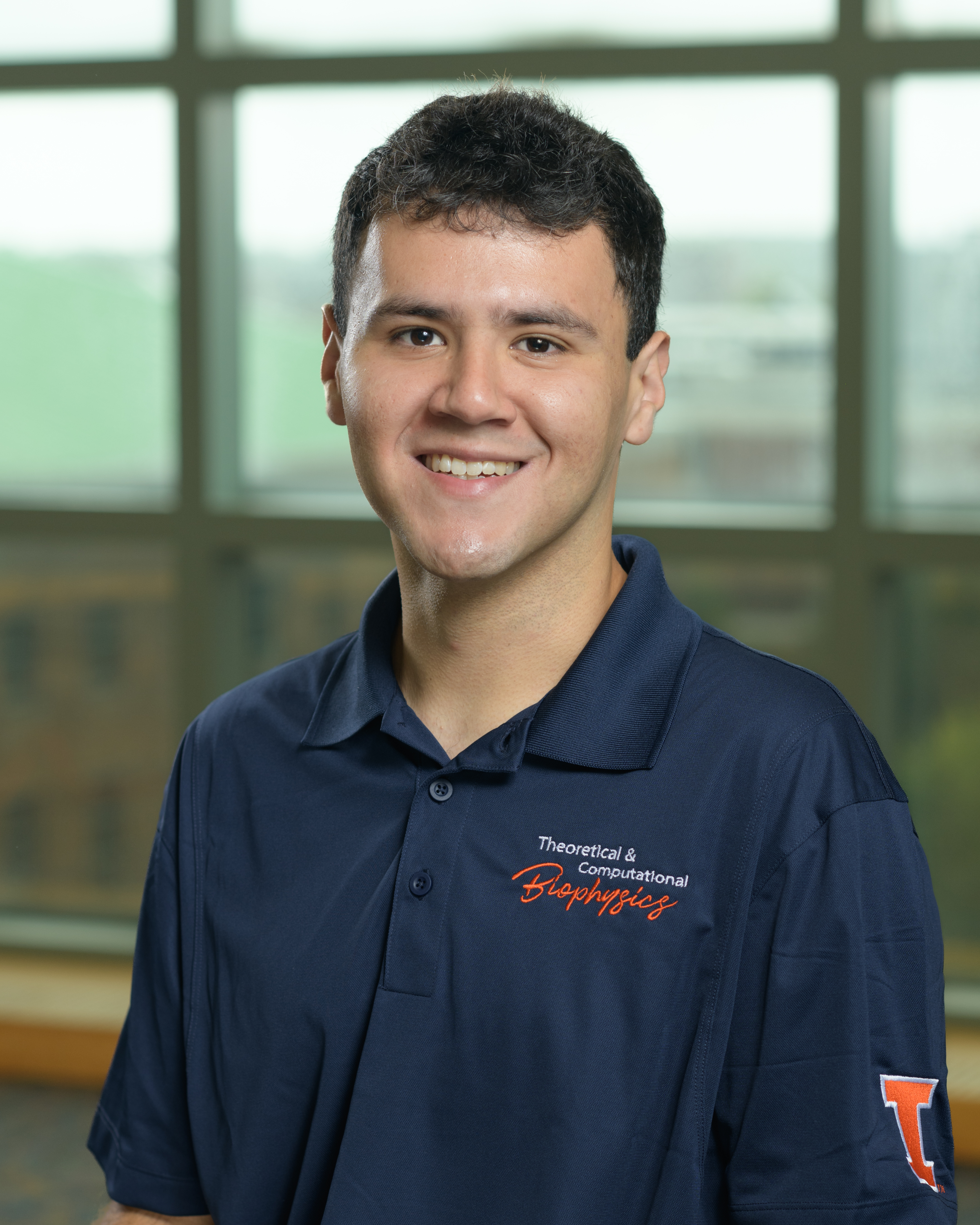
Research Interests
-
Machine Learning as a Biophysical Reaction Coordinate and Structure Prediction and Analysis Tool
-
Machine Learning Aided Coarse Grained and Molecular Dynamics Simulations
-
Machine Learning in Biophysics and Statistical Physics
-
Development of Biochemically / Biophysically Driven Deep Learning Networks