TCBG Seminar
Identifying informative distance measures in high-dimensional feature spaces Tweet
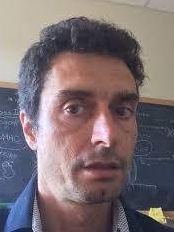
Professor
Alessandro Laio
Statistical and Biological Physics
SISSA International School for Advanced Studies
Trieste, Italy
Friday, October 8, 2021
10:00 am (CT)
Zoom webinar recording
Abstract
Real-world data in biochemistry, material science and beyond typically contain a large number of features that are often heterogeneous in nature, relevance, and also units of measure. When assessing the similarity between data points, one can build various distance measures using subsets of these features. Finding a small set of features that still retains sufficient information about the dataset is important for the successful application of many statistical learning approaches. We introduce a statistical test that can assess the relative information retained when using two different distance measures, and determine if they are equivalent, independent, or if one is more informative than the other. This ranking can in turn be used to identify the most informative distance measure and, therefore, the most informative set of features, out of a pool of candidates. The approach is applied to find the most relevant policy variables for controlling the Covid-19 epidemic and to identify compact yet informative descriptors for atomic structures. We further provide evidence that the information asymmetry measured by the proposed test can be used to infer relationships of causality between the features of a dataset.